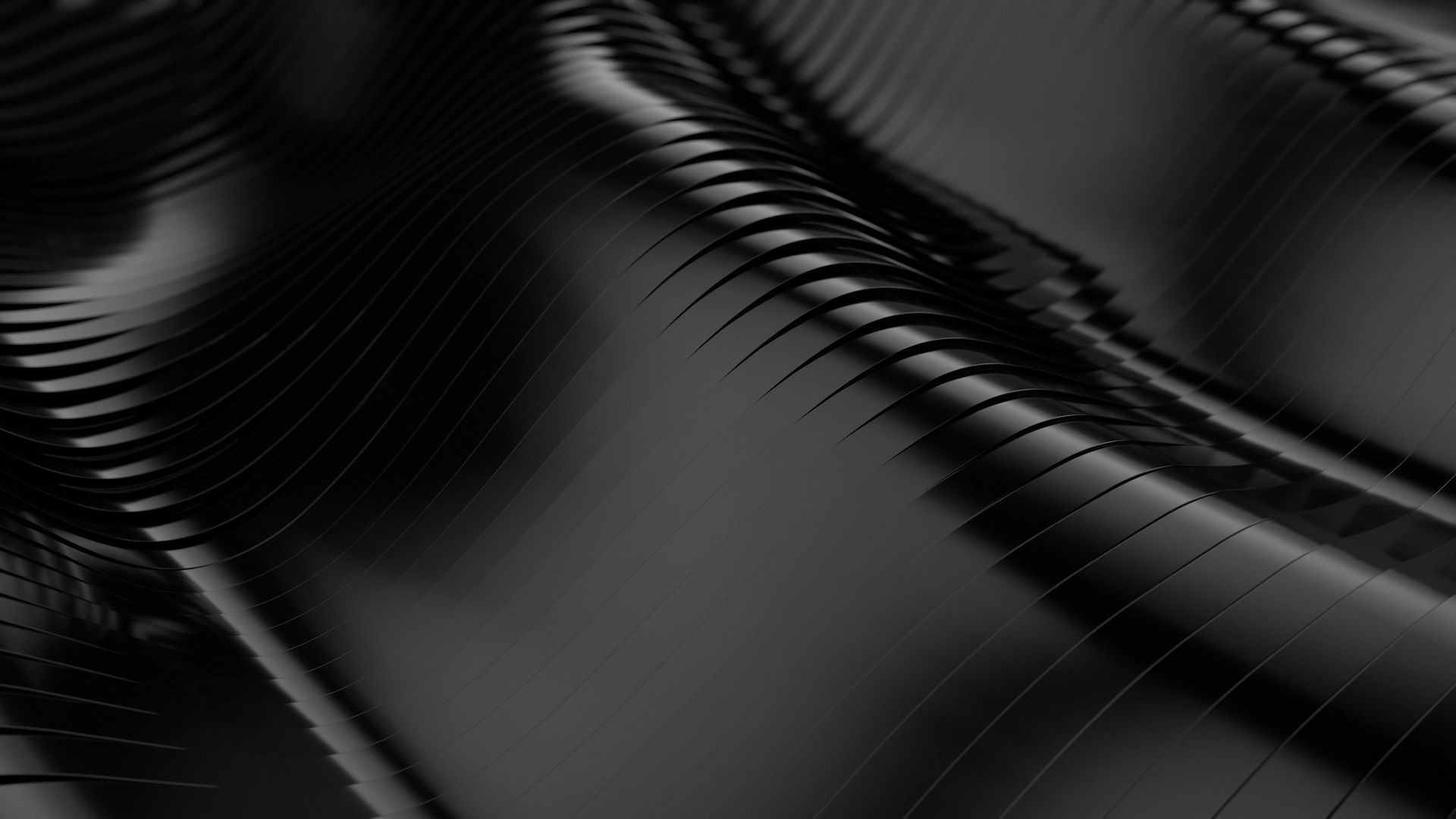
Classification (Tensorflow)
Summary
Building custom models for classification problem with Tensorflow.
Example 1. Two labels using Binary Crossentropy loss function.
import numpy as np
import pandas as pd
import matplotlib.pyplot as plt
import seaborn as sn
import tensorflow as tf
### Creating data.
from sklearn.datasets import make_circles
n_samples = 1200
X, y = make_circles(n_samples, noise=0.03, random_state=42)
circles = pd.DataFrame({"X0":X[:, 0], "X1":X[:, 1], "label":y})
plt.scatter(X[:, 0], X[:, 1], c=y, cmap=plt.cm.RdYlBu)
### Split data into train, valditaion and test sets.
X_train, y_train = X[:800], y[:800]
X_val, y_val = X[800:1000], y[800:1000]
X_test, y_test = X[1000:1200], y[1000:1200]
### model.
tf.random.set_seed(42)
model = tf.keras.Sequential([tf.keras.layers.Dense(4, activation=tf.keras.activations.relu),
tf.keras.layers.Dense(4, activation=tf.keras.activations.relu),
tf.keras.layers.Dense(1, activation=tf.keras.activations.sigmoid)])
model.compile(loss=tf.keras.losses.binary_crossentropy,
optimizer=tf.keras.optimizers.Adam(lr=0.01),
metrics=['accuracy'])
history = model.fit(X_train, y_train, validation_data=(X_val,y_val), verbose=1, epochs=25)
### Evaluate the model.
df = pd.DataFrame(history.history)
fig1,ax1 = plt.subplots(1,2)
ax1[0].plot(df['loss'], label='train loss')
ax1[0].plot(df['val_loss'], label='validation loss')
ax1[0].set_xlabel('epoch')
ax1[0].set_ylabel('loss')
ax1[0].set_title('Model loss curves')
ax1[0].legend()
ax1[0].grid()
ax1[1].plot(df['accuracy'], label='train accuracy')
ax1[1].plot(df['val_accuracy'], label='validation accuracy')
ax1[1].set_xlabel('epoch')
ax1[1].set_ylabel('accuracy')
ax1[1].set_title('Model accuracy curves')
ax1[1].legend()
ax1[1].grid()
loss, accuracy = model.evaluate(X_test, y_test)
print(f"Model loss on the test set: {loss:.5f}") # Model loss on the test set: 0.05795
print(f"Model accuracy on the test set: {100*accuracy:.2f}%") # Model accuracy on the test set: 99.50%
### Make predictions.
y_preds = model.predict(X_test)
### Confusion Matrix.
df = pd.DataFrame({'True':y_test,
'Prediction':tf.round(tf.squeeze(y_preds).numpy())})
confusion_matrix = pd.crosstab(df['True'], df['Prediction'], rownames=['True'], colnames=['Prediction'])
sn.heatmap(confusion_matrix, annot=True, fmt=".1f")
### Plot the decision boundaries for the training and test sets.
def plot_decision_boundary(model, X, y):
# Define the axis boundaries of the plot and create a meshgrid.
x_min, x_max = X[:, 0].min() - 0.1, X[:, 0].max() + 0.1
y_min, y_max = X[:, 1].min() - 0.1, X[:, 1].max() + 0.1
xx, yy = np.meshgrid(np.linspace(x_min, x_max, 100),
np.linspace(y_min, y_max, 100))
# Create X values (we're going to predict on all of these).
x_in = np.c_[xx.ravel(), yy.ravel()]
# Make predictions using the trained model.
y_pred = model.predict(x_in)
y_pred = np.round(y_pred).reshape(xx.shape)
# Plot decision boundary.
plt.contourf(xx, yy, y_pred, cmap=plt.cm.RdYlBu, alpha=0.7)
plt.scatter(X[:, 0], X[:, 1], c=y, s=40, cmap=plt.cm.RdYlBu)
plt.xlim(xx.min(), xx.max())
plt.ylim(yy.min(), yy.max())
plt.figure(figsize=(12, 6))
plt.subplot(1, 2, 1)
plt.title("Train")
plot_decision_boundary(model, X=X_train, y=y_train)
plt.subplot(1, 2, 2)
plt.title("Test")
plot_decision_boundary(model, X=X_test, y=y_test)



